Data Cleaning: Ensuring Data Accuracy for Informed Decisions
In today’s data-driven world, accurate and reliable information is crucial for making informed decisions. This is especially true in various industries, including real estate, finance, healthcare, and marketing, where data plays a pivotal role in strategizing and achieving success. However, raw data is often messy and prone to errors, which can lead to incorrect conclusions and faulty decisions. Data cleaning, also known as data cleansing or data scrubbing, is the process of identifying and correcting errors, inconsistencies, and inaccuracies in datasets. In this blog post, we will explore the importance of data cleaning, its key benefits, and how it ensures data accuracy for making informed decisions.
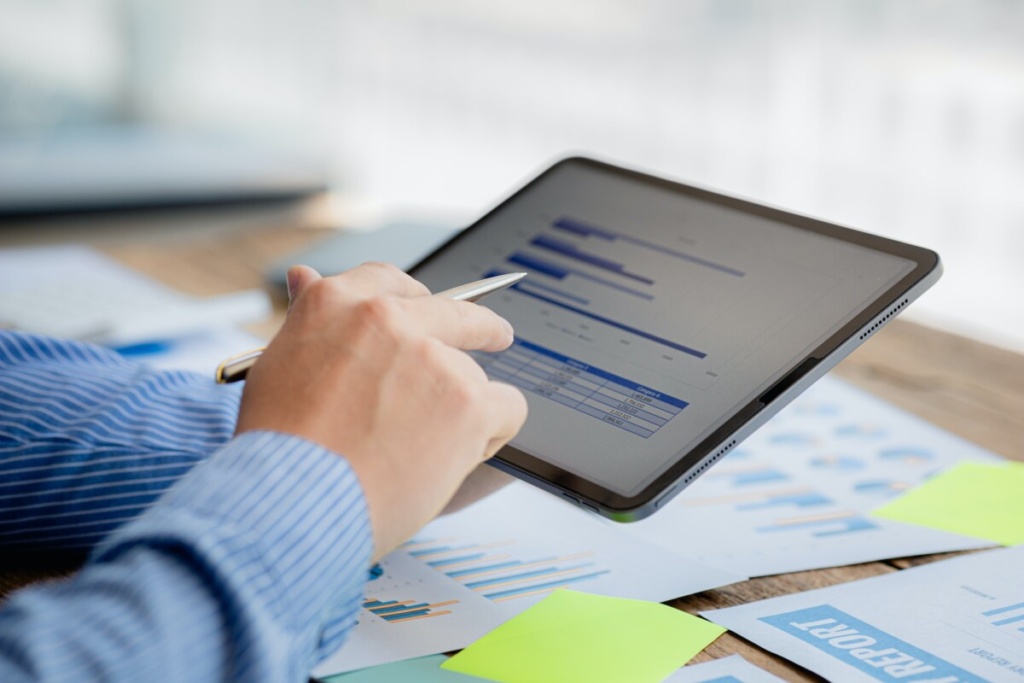
Understanding Data Cleaning
Data cleaning is an essential step in the data analysis process. It involves detecting and rectifying errors, duplications, and inconsistencies in datasets to improve data quality. Data can become corrupt due to various factors, such as human errors during data entry, system glitches, data integration issues, and missing values. Data cleaning addresses these challenges and transforms raw data into accurate and reliable information for analysis and decision-making.
Importance of Data Cleaning
Enhancing Data Quality
Data cleaning significantly improves the quality of data. By identifying and rectifying errors, duplications, and inconsistencies, organizations can trust the accuracy of their data for decision-making processes.
Reliable Insights
Clean data leads to reliable insights and conclusions. Decision-makers can confidently base their strategies on accurate data, reducing the risk of making faulty decisions that could have far-reaching consequences.
Efficient Data Analysis
Data cleaning streamlines the data analysis process. Analysts spend less time dealing with errors and more time on meaningful analysis, allowing for faster and more efficient decision-making.
Building Trust
Accurate data builds trust among stakeholders, clients, and partners. When organizations can demonstrate the reliability of their data, they gain credibility and foster stronger relationships with their stakeholders.
Minimizing Costs
Data errors can be costly. From making inaccurate investments to launching ineffective marketing campaigns, faulty data can lead to wasted resources. Data cleaning prevents such losses by ensuring that resources are allocated wisely based on accurate information.
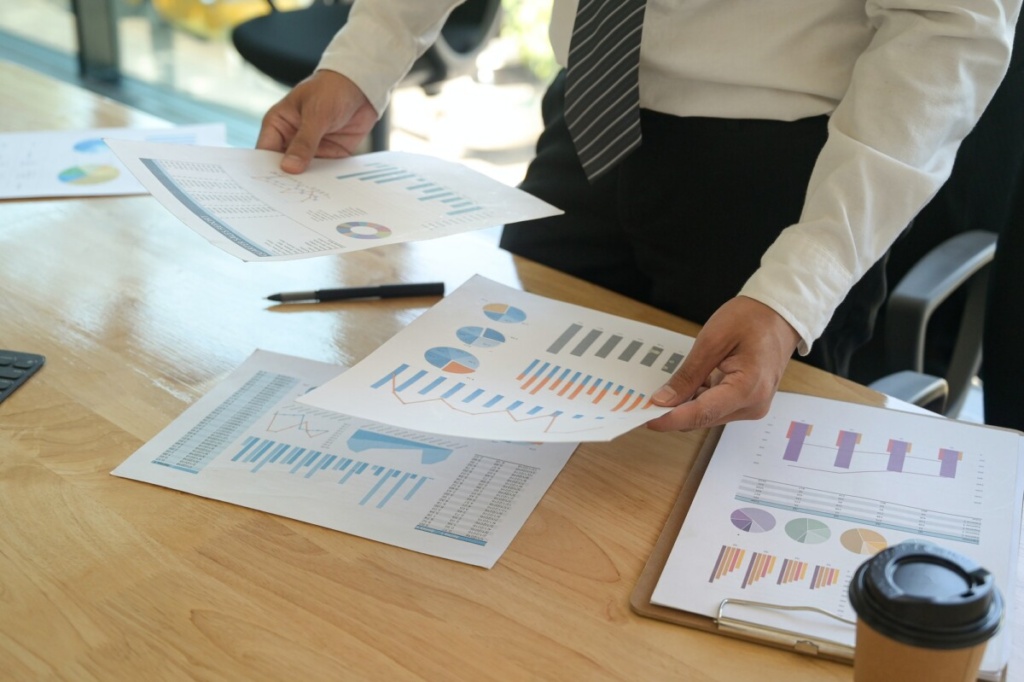
Key Steps in Data Cleaning
Data Profiling: Data profiling involves assessing the quality and structure of the dataset. It helps identify patterns, outliers, and potential errors that need cleaning.
Data Standardization: Standardizing data ensures consistency and uniformity. This step includes converting data into a common format and eliminating variations, such as abbreviations or misspellings.
Removing Duplicates: Duplicated data can lead to skewed analysis and conclusions. Removing duplicates ensures that each data point is unique and accurate.
Handling Missing Values: Missing data can affect analysis and decision-making. Data cleaning involves strategies to handle missing values, such as imputation or removing incomplete records.
Validating Data: Data validation involves checking data against predefined rules or constraints to ensure accuracy and consistency.
Outlier Detection: Outliers are extreme values that can distort analysis. Data cleaning includes detecting and addressing outliers appropriately.
Cross-Referencing Data: Cross-referencing data with trusted sources can verify the accuracy of the information and identify potential errors.
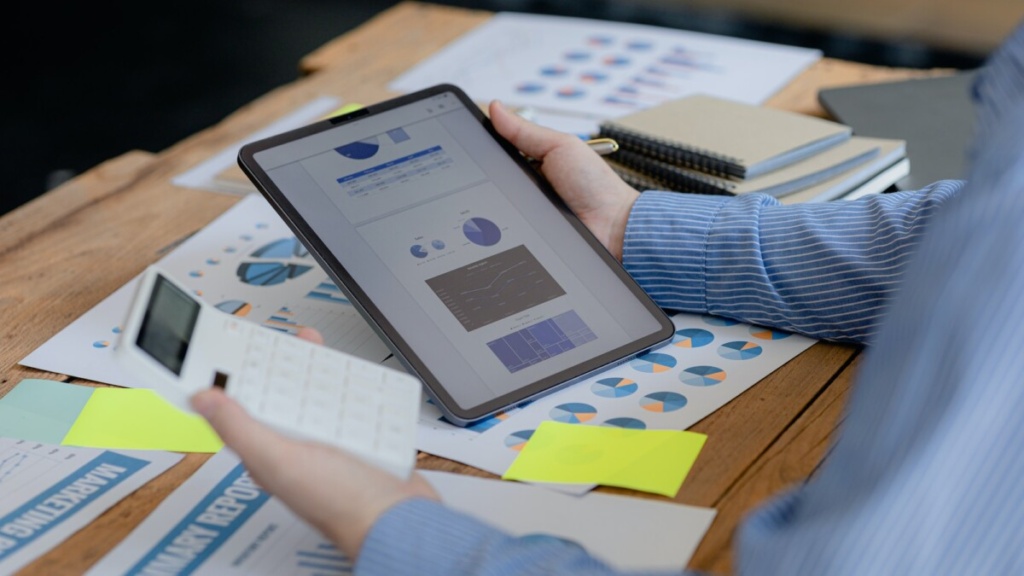
Benefits of Data Cleaning
Accurate Decision-Making
Data cleaning ensures that decisions are based on accurate and reliable information, reducing the risk of making faulty judgments.
Improved Business Performance
With clean data, organizations can develop effective strategies, optimize processes, and drive better business performance.
Enhanced Customer Experience
Accurate data enables organizations to understand customer behavior and preferences better, leading to more personalized and satisfying customer experiences.
Compliance and Risk Mitigation
Data cleaning helps organizations adhere to data protection regulations and reduces the risk of legal and financial repercussions due to data errors.
Increased Efficiency
Clean data streamlines operations, leading to improved efficiency, reduced operational costs, and increased productivity.
Data Cleaning Tools and Technologies
Several tools and technologies facilitate data cleaning processes. Some popular ones include:
OpenRefine: OpenRefine is an open-source tool that offers powerful data cleaning and transformation capabilities.
Trifacta: Trifacta is a data wrangling platform that automates the data cleaning process using machine learning algorithms.
Microsoft Excel: Excel offers various data cleaning functionalities, such as removing duplicates, handling missing values, and data standardization.
Python and R: Python and R programming languages provide numerous libraries and packages for data cleaning tasks.
Conclusion
Data cleaning is a critical process for ensuring data accuracy and reliability. In the data-driven era, organizations must make informed decisions based on trustworthy information. By investing in data cleaning, businesses can enhance data quality, improve decision-making, and drive better outcomes. Data cleaning is not a one-time task but an ongoing process, as data continuously evolves. Organizations that prioritize data cleaning will gain a competitive advantage, build trust among stakeholders, and position themselves for long-term success. As the importance of data-driven decisions continues to grow, data cleaning becomes an indispensable practice for any organization seeking excellence in their operations and strategic endeavors.