Unlocking the Power of Predictive Analytics
In the fast-paced world of real estate, data-driven insights are becoming increasingly essential for making strategic decisions. As the industry evolves, real estate professionals are turning to data science and predictive analytics to gain a competitive edge. Predictive analytics, a powerful branch of data science, allows stakeholders to harness the potential of historical data and advanced algorithms to anticipate market trends and optimize investment strategies. In this blog post, we will delve deeper into the role of data science in real estate, explore the applications of predictive analytics, and understand how it empowers stakeholders to make informed choices.
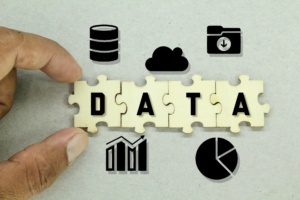
The Role of Data Science in Real Estate
Data science has transformed the way the real estate industry operates, providing invaluable insights and improving decision-making processes. Some of the key roles data science plays in real estate are:
1. Market Analysis: Data science enables professionals to conduct comprehensive market analyses by analyzing historical data, identifying trends, and forecasting future conditions. This allows them to gain a better understanding of market dynamics and make strategic decisions accordingly.
2. Customer Behavior Analysis: By leveraging data science techniques, real estate professionals can segment customers based on their preferences and buying behaviors. This segmentation helps tailor marketing strategies to specific customer groups, resulting in better engagement and conversion rates.
3. Risk Mitigation: Data science aids in assessing investment risks by analyzing factors such as market volatility, economic indicators, and external influences. This empowers stakeholders to take proactive measures to minimize potential risks.
4. Price Prediction: Predictive analytics models can accurately forecast property prices based on historical data and market trends. This information is invaluable for both buyers and sellers, allowing them to negotiate better deals and make data-driven pricing decisions.
5. Optimized Marketing: Data science helps optimize marketing efforts by analyzing customer data and behavior. By understanding what resonates with their target audience, real estate professionals can create targeted campaigns and maximize returns on their marketing investments.
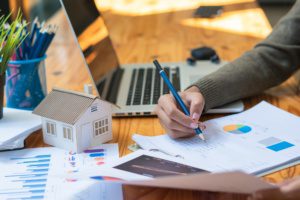
Predictive Analytics Applications in Real Estate
1. Price Forecasting: Predictive analytics models can accurately forecast future property prices based on historical data, location, economic indicators, and other relevant factors. This helps buyers and sellers make well-informed decisions.
2. Demand Prediction: Predictive models can estimate future market demand for specific property types or locations. This empowers developers to focus on areas with high demand and tailor their projects accordingly.
3. Investment Analysis: Data science allows investors to evaluate the potential return on investment (ROI) for a property. By considering factors such as purchase price, rental income, expenses, and appreciation, investors can make data-driven decisions about their portfolios.
4. Tenant Turnover Forecasting: For property managers, predictive models can forecast the likelihood of tenant turnover. This allows them to proactively address potential vacancies and plan for maintenance and leasing activities.
5. Time-on-Market Estimation: Predictive models can estimate the time a property is likely to spend on the market before being sold. This information helps sellers plan their marketing strategies and manage their expectations.
The Data Science Process for Real Estate
1. Data Collection: Gather relevant data from various sources, including MLS databases, public records, economic indicators, and customer interactions.
2. Data Cleaning and Preprocessing: Ensure data accuracy and consistency by removing duplicates, handling missing values, and correcting errors.
3. Feature Engineering: Select and engineer relevant features (variables) from the data to improve the predictive models accuracy.
4. Model Selection: Choose the appropriate predictive modeling technique, such as linear regression, decision trees, or neural networks, based on the nature of the problem and the data.
5. Model Training: Train the predictive model using historical data, optimizing its parameters to achieve the best performance.
6. Model Validation: Validate the predictive model using a separate set of data to ensure its accuracy and generalization capability.
7. Model Deployment: Deploy the trained model to make predictions on new data and incorporate it into real estate operations.
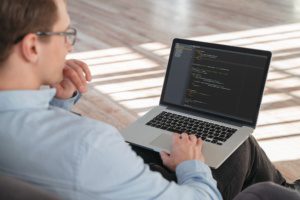
Tools and Technologies for Real Estate Data Science
1. Python: A popular programming language for data science, offering numerous libraries for data analysis and predictive modeling, such as Pandas, NumPy, and Scikit-learn.
2. R: Another widely used programming language for data analysis and statistical modeling, with a rich ecosystem of packages like ggplot2 and caret.
3. TensorFlow and Keras: Libraries for building and deploying machine learning models, including deep learning neural networks.
4. Microsoft Azure Machine Learning: A cloud-based platform that facilitates data science tasks, including data preparation, modeling, and deployment.
5. Tableau and Power BI: Data visualization tools that can help real estate professionals gain actionable insights from their data.
Case Study: Real Estate Investment and Predictive Modeling
Investor XYZ: A real estate investor aiming to optimize their investment decisions.
Predictive Model Implementation: Investor XYZ implemented predictive modeling to analyze historical property data, economic indicators, and neighborhood characteristics. The model was trained to forecast future property prices and identify areas with high growth potential.
Results:
1. Smart Investment Choices: Equipped with predictive insights, Investor XYZ made strategic investments in locations projected to experience substantial appreciation, leading to higher ROI.
2. Risk Mitigation: The predictive model helped Investor XYZ identify potential risks, such as areas with declining property values, enabling them to avoid risky investments.
3. Data-Driven Decision-Making: By leveraging predictive modeling, Investor XYZ was able to make data-driven decisions rather than relying solely on gut instincts or market rumors.
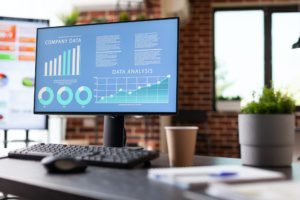
Conclusion
Data science and predictive analytics are transforming the real estate industry, providing real estate professionals with the tools they need to make data-driven decisions and anticipate market trends. By analyzing historical data, understanding customer behavior, and forecasting future market conditions, stakeholders can gain a competitive edge and achieve success in their endeavors. The integration of data science into real estate operations is no longer a luxury; it has become a necessity for sustained growth and profitability. Leveraging predictive models, real estate professionals can navigate the ever-changing market landscape with confidence, making strategic choices that lead to smart investments and unparalleled success. With data science as their guiding force, the future of real estate is brimming with possibilities, where informed decisions shape the path to prosperity.
[…] Unlocking the Power of Predictive AnalyticsIn the fast-paced world of real estate, data-driven insights are becoming increasingly essential for making strategic decisions. As the industry evolves, real estate professionals are turning to data science and predictive analytics to gain a… […]