Predictive Models in Real Estate: Forecasting Property Prices
In the fast-paced world of real estate, accurately forecasting property prices is a game-changer. The ability to predict future price movements allows real estate professionals, investors, and buyers to make informed decisions and seize profitable opportunities. This is where predictive models come into play. Predictive models in real estate leverage historical data and advanced algorithms to forecast property prices, enabling stakeholders to navigate the market strategically. In this blog post, we will delve into the world of predictive models in real estate and understand their significance in forecasting property prices.
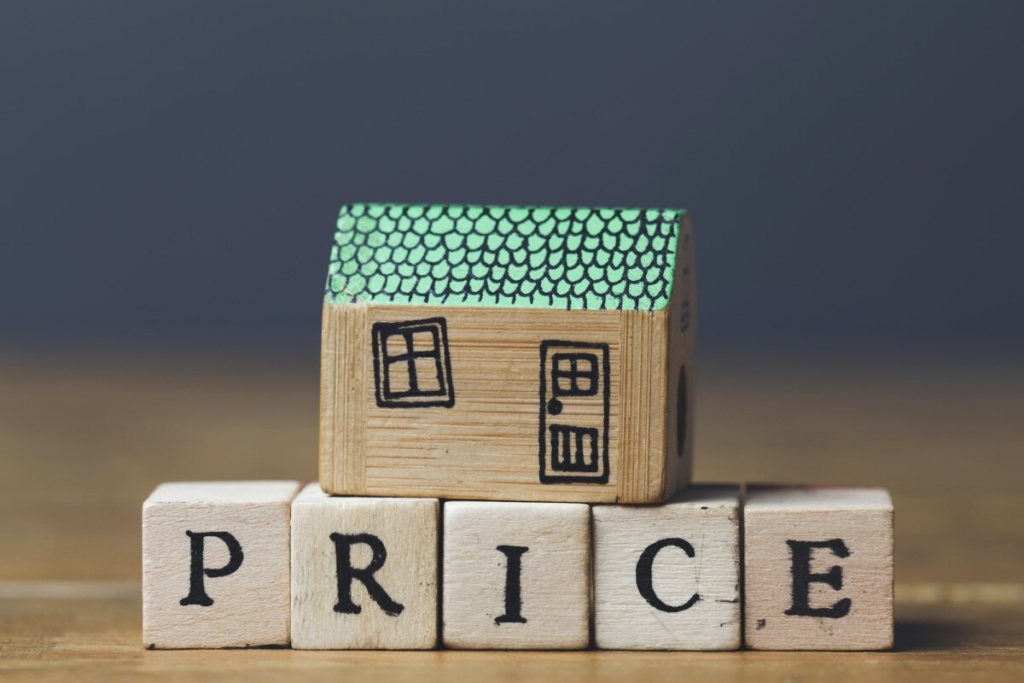
The Role of Predictive Models in Real Estate
Predictive models are mathematical algorithms that analyze historical data and identify patterns to make predictions about future events. In the context of real estate, predictive models analyze factors such as property characteristics, market trends, economic indicators, and demographic data to forecast property prices. These models can provide insights into property price trends, market fluctuations, and investment opportunities.
The key benefits of using predictive models in real estate include:
1. Informed Decision-Making: Predictive models enable real estate professionals and investors to make data-driven decisions, reducing risks and maximizing returns.
2. Market Insights: Predictive models provide valuable insights into market trends and dynamics, helping stakeholders understand supply and demand dynamics in different neighborhoods.
3. Identifying Investment Opportunities: Predictive models can identify potentially undervalued properties or emerging markets, guiding investors towards profitable opportunities.
4. Pricing Strategies: For real estate developers and sellers, predictive models assist in setting optimal property prices based on market conditions and demand.
5. Risk Mitigation: By analyzing historical data and patterns, predictive models can help identify potential risks, allowing stakeholders to take proactive measures.
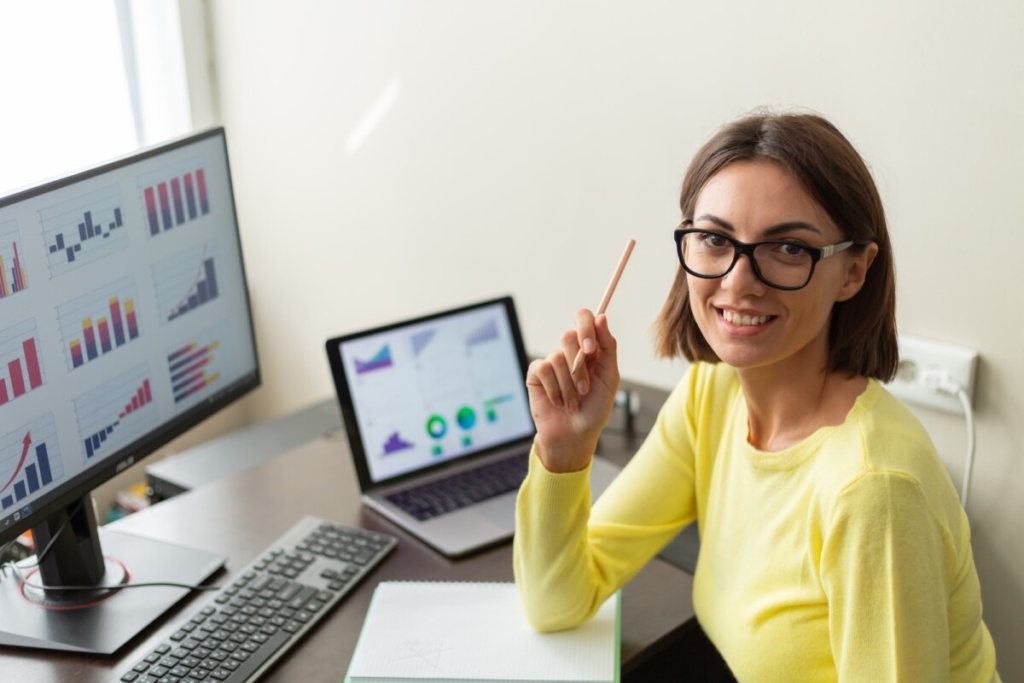
Types of Predictive Models in Real Estate
1. Multiple Regression Analysis: This model examines the relationship between property prices and various predictor variables, such as location, square footage, number of bedrooms, and other property characteristics.
2. Time Series Analysis: Time series analysis is used to forecast property prices based on historical price data, considering seasonality and trends over time.
3. Machine Learning Algorithms: Machine learning algorithms, such as decision trees, random forests, and neural networks, are increasingly utilized for more complex predictive modeling in real estate.
4. Hedonic Pricing Model: The hedonic pricing model estimates the value of different property attributes and how they contribute to the overall property price.
Data Requirements for Predictive Models in Real Estate
Predictive models rely on historical data for training and validation. To build accurate predictive models in real estate, the following data types are essential:
1. Historical Property Transactions: Information on past property sales, including sale prices, property characteristics, and transaction dates.
2. Market Trends: Data on market trends, such as inventory levels, days on market, and supply-demand dynamics.
3. Economic Indicators: Data on economic factors, such as interest rates, employment rates, and GDP growth, which can influence property prices.
4. Demographic Data: Demographic information, such as population density and household income, can provide insights into local market preferences and demand.
5. Property Characteristics: Detailed data on property characteristics, including size, location, amenities, and condition.
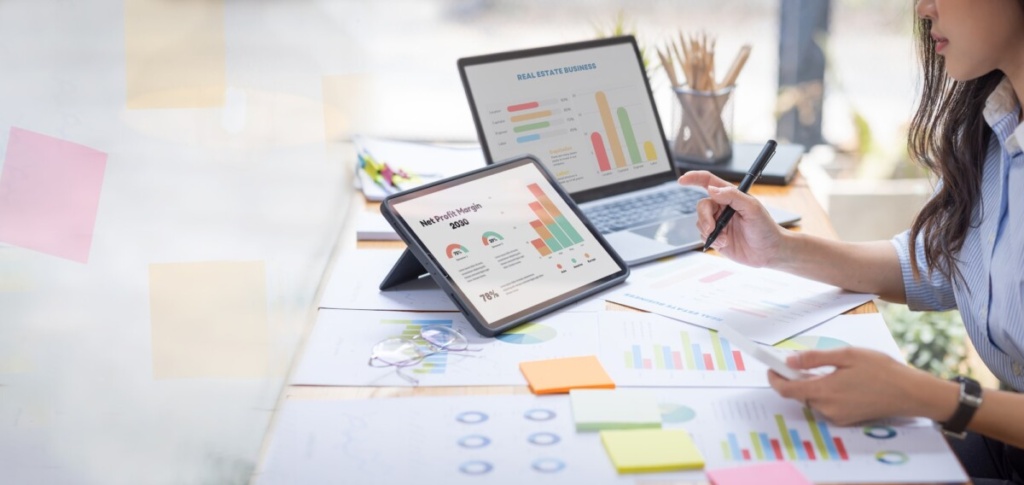
Challenges in Predictive Modeling for Real Estate
While predictive models offer valuable insights, they come with their challenges:
1. Data Quality: Accurate and reliable data is essential for building robust predictive models. Data quality issues can lead to inaccurate predictions.
2. Overfitting: Overfitting occurs when a model is too complex and performs well on the training data but poorly on new data. It is crucial to strike a balance between model complexity and performance.
3. Data Availability: Availability of data can vary depending on the region and property type, making it challenging to build predictive models in certain markets.
4. Market Volatility: Real estate markets can be influenced by unpredictable external factors, such as economic changes and policy shifts, which can affect the accuracy of predictions.
Real-Life Example of Predictive Models in Real Estate
A real estate investment firm is interested in predicting property price trends in a particular city. They gather historical data on property transactions, market trends, and demographic information for the past ten years. Using machine learning algorithms, the firm builds a predictive model that takes into account property characteristics, market trends, and economic indicators.
The predictive model analyzes the data and forecasts property price trends for the next few years. Based on the model’s insights, the investment firm identifies neighborhoods with potential for growth and plans its investment strategy accordingly.
Conclusion
Predictive models in real estate offer a powerful tool for forecasting property prices and making informed decisions in a dynamic market. By analyzing historical data and leveraging advanced algorithms, predictive models provide valuable insights into market trends, investment opportunities, and pricing strategies.
While challenges exist in building predictive models, advancements in technology and data analytics continue to enhance their accuracy and reliability. As real estate professionals and investors navigate the ever-changing landscape of the real estate market, predictive models will remain instrumental in identifying opportunities, reducing risks, and maximizing returns.
In the data-driven world of real estate, predictive models stand as a critical asset, guiding stakeholders towards success and empowering them to make strategic decisions that lead to remarkable achievements in the dynamic realm of property investments.