Using Machine Learning in Real Estate: Predicting Rental Demand
Machine Learning (ML) is transforming various industries, and the real estate sector is no exception. ML algorithms offer valuable predictive capabilities that can revolutionize rental property management and investment strategies. In this blog post, we will explore the application of Machine Learning in real estate, focusing on predicting rental demand. By harnessing data-driven insights, real estate professionals can make informed decisions, optimize rental property investments, and enhance overall portfolio performance.
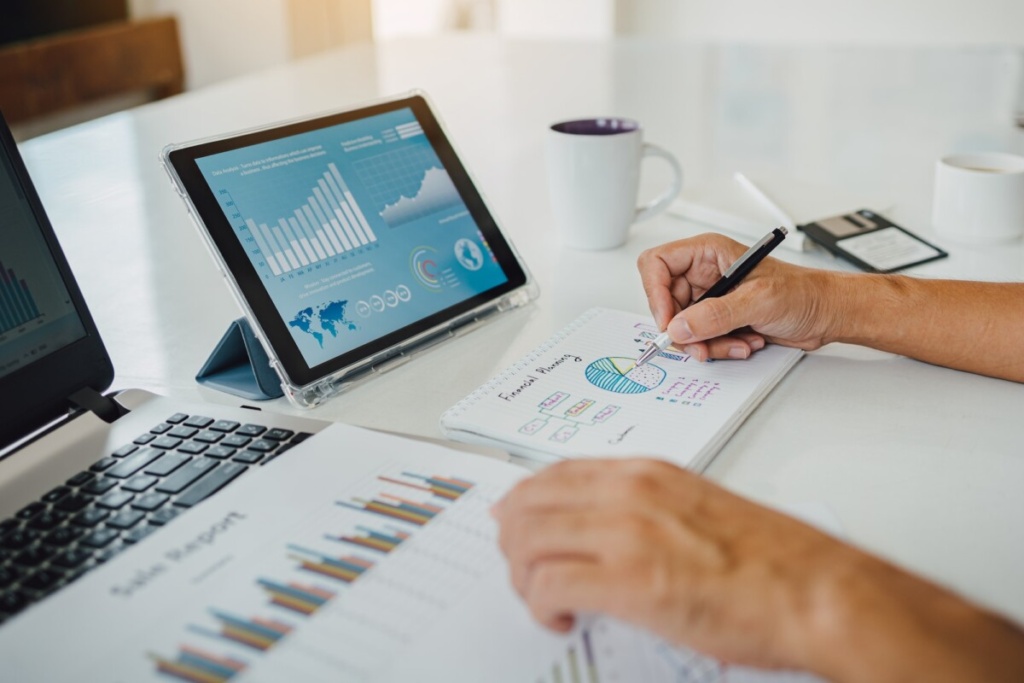
Understanding Machine Learning in Real Estate
Machine Learning is a subset of Artificial Intelligence (AI) that enables computer systems to learn from data without explicit programming. In the context of real estate, ML algorithms analyze historical rental data, property characteristics, demographic information, and market trends to identify patterns and make predictions.
Predicting Rental Demand with Machine Learning
Data Collection and Preprocessing:
To predict rental demand accurately, real estate professionals need relevant and clean data. This data includes historical rental transaction records, property features (e.g., location, size, amenities), economic indicators, and demographic information. Data preprocessing involves cleaning and organizing the data to ensure its quality and consistency.
Feature Engineering:
Feature engineering is a critical step in preparing the data for ML algorithms. It involves selecting and transforming relevant features that will impact rental demand predictions. For instance, factors such as proximity to public transportation, schools, and job centers could significantly influence rental demand in a specific area.
Model Selection:
Choosing the right ML model is crucial for accurate predictions. Commonly used models for predicting rental demand include Linear Regression, Decision Trees, Random Forests, and Gradient Boosting algorithms. Each model has its strengths and is suitable for different scenarios.
Training the Model:
During the training phase, the ML model learns from the historical data to identify patterns and relationships between features and rental demand. The data is divided into a training set and a testing set to evaluate the model’s performance.
Validation and Optimization:
After training the model, it needs to be validated and optimized to ensure its accuracy. Cross-validation techniques help assess the model’s performance on different subsets of the data, reducing the risk of overfitting.
Making Predictions:
Once the model is validated and optimized, it can be used to make predictions on new data. Real estate professionals can input property characteristics and market data to forecast rental demand in specific areas or for particular properties.
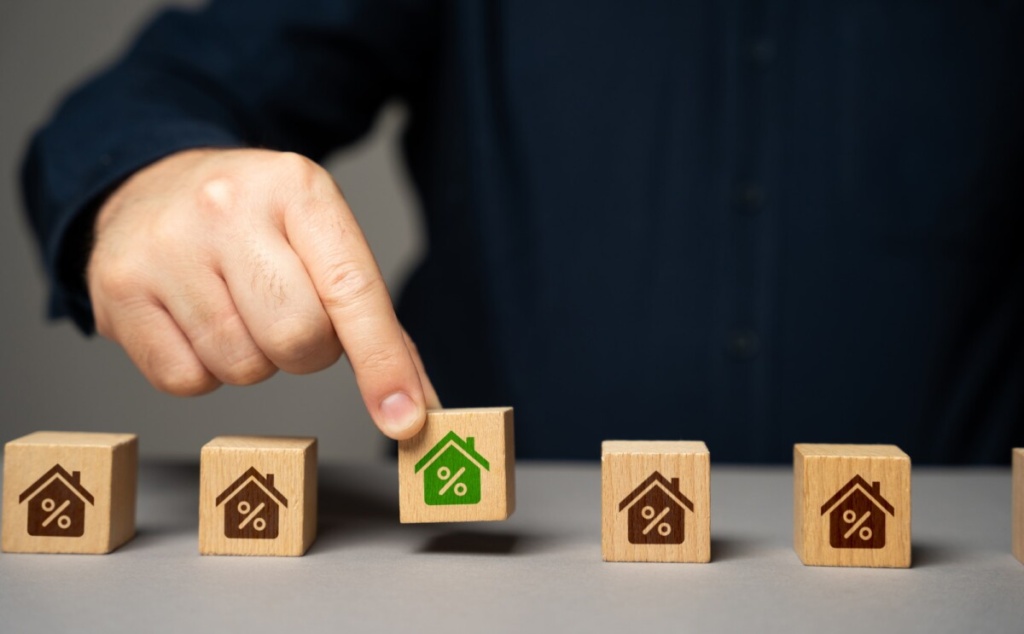
Benefits of Predicting Rental Demand with Machine Learning
Informed Investment Decisions:
Accurate rental demand predictions enable real estate investors to identify high-demand areas, leading to more profitable investment decisions.
Optimal Pricing Strategies:
Machine Learning helps set competitive rental prices by considering demand trends and property attributes, maximizing rental income while attracting tenants.
Vacancy Reduction:
Predicting rental demand allows property owners to proactively market vacancies and reduce the time properties remain unoccupied.
Personalized Marketing:
Real estate professionals can tailor marketing efforts to potential tenants based on predicted demand, improving lead generation and tenant matching.
Portfolio Diversification:
Machine Learning insights facilitate diversification strategies by identifying emerging rental markets and areas with growth potential.
Conclusion
Machine Learning is reshaping the real estate industry, empowering professionals to predict rental demand with precision. By leveraging data-driven insights, real estate investors, developers, and property managers can make informed decisions, optimize rental property investments, and enhance overall portfolio performance. As ML algorithms continue to advance, the accuracy of rental demand predictions will improve, contributing to a more efficient, profitable, and customer-centric real estate market.